We solve algorithmic, modeling and computational problems in AI, machine learning, quantum computing, network science, graphs, combinatorial scientific computing and more.
Friday, May 15, 2020
Our work in HPCwire
Argonne Receives Two Awards from DARPA for Quantum Information Science
Tuesday, April 21, 2020
NSF grant to tackle COVID-19
Clemson news coverage: Artificial intelligence could aid in fight against COVID-19
Ilya Safro of @socclemson said that his team will soon roll out a new artificial intelligence system aimed at helping researchers explore the scientific literature as they strive for new discoveries to combat the novel coronavirus.https://t.co/TwQyZqEUra pic.twitter.com/fh3K8iAoWO
— Clemson Engineering, Computing & Applied Sciences (@ClemsonCECAS) April 21, 2020
Tuesday, April 14, 2020
Board game night
Board game night from quarantine! pic.twitter.com/6env2hICaa
— Justin Sybrandt, Ph.D. (@Justin_Sybrandt) March 28, 2020
Monday, April 13, 2020
Friday, April 3, 2020
From SoC@Clemson
Congrats to Dr. Ilya Safro on receiving funding from @DARPA to work on hybrid quantum-classical algorithms for combinatorial optimization problems, a 4 year collaborative research with @argonne. He is looking for Ph.D. students to join the Algorithms & Computational Science lab. pic.twitter.com/YfIYVc7HzO
— School of Computing (@socclemson) April 3, 2020
Thursday, March 26, 2020
PhD thesis defense
Congratulations to Dr. Justin Sybrandt for successfully defending his Ph.D. thesis "Exploiting Latent Features of Text and Graphs"! Justin will join Google Brain this summer.
Wednesday, February 19, 2020
New papers on biomedical NLP and hypothesis generation
New papers on biomedical NLP+hypothesis generation.
Sybrandt, Safro "CBAG: Conditional Biomedical Abstract Generation", http://arxiv.org/pdf/2002.05637.pdf,
Sybrandt, Tyagin, Shtutman, Safro "AGATHA: Automatic Graph-mining and Transformer based Hypothesis Generation" http://arxiv.org/pdf/2002.05635.pdf
Friday, January 17, 2020
Congratulations to PhD student Ruslan Shaydulin
Congratulations to PhD student Ruslan Shaydulin, advised by Dr. Ilya Safro, for receiving 2020 Maria Goeppert Mayer fellowship for postdoctoral studies at Argonne National Lab. Ruslan will start in Fall 2020. He was selected among many candidates from top schools and different disciplines.
Saturday, October 19, 2019
How to find the best location for wireless charging lanes
Our paper is accepted in Journal of Industrial Management and Optimization
Ushijima-Mwesigwa, Khan, Chowdhury, Safro "Optimal Installation for Electric Vehicle Wireless Charging Lanes", 2019
The emergence of electric vehicle wireless charging technology, where a whole lane can be turned into a charging infrastructure, leads to new challenges in the design and analysis of road networks. From a network perspective, a major challenge is determining the most important nodes with respect to the placement of the wireless charging lanes. In other words, given a limited budget, cities could face the decision problem of where to place these wireless charging lanes. With a heavy price tag, a placement without a careful study can lead to inefficient use of limited resources. In this work, the placement of wireless charging lanes is modeled as an integer programming problem. The basic formulation is used as a building block for different realistic scenarios. We carry out experiments using real geospatial data and compare our results to different network-based heuristics.
Reproducibility: all datasets, algorithm implementations and mathematical programming formulation presented in this work are available at https://github.com/hmwesigwa/smartcities.git
Monday, October 14, 2019
Wednesday, September 25, 2019
Multistart Methods for Quantum Approximate Optimization
Best student paper award at IEEE HPEC2019 goes to our lab! Congratulations to the leading student Ruslan Shaydulin!
Ruslan Shaydulin, Ilya Safro, Jeffrey Larson "Multistart Methods for Quantum Approximate Optimization", accepted at IEEE High Performance Extreme Computing Conference (HPEC) 2019, preprint at https://arxiv.org/abs/1905.08768
Hybrid quantum-classical algorithms such as the quantum approximate optimization algorithm (QAOA) are considered one of the most promising approaches for leveraging near-term quantum computers for practical applications. Such algorithms are often implemented in a variational form, combining classical optimization methods with a quantum machine to find parameters to maximize performance. The quality of the QAOA solution depends heavily on quality of the parameters produced by the classical optimizer. Moreover, multiple local optima in the space of parameters make it harder for the classical optimizer. In this paper we study the use of a multistart optimization approach within a QAOA framework to improve the performance of quantum machines on important graph clustering problems. We also demonstrate that reusing the optimal parameters from similar problems can improve the performance of classical optimization methods, expanding on similar results for MAXCUT.
Thursday, June 20, 2019
The best of both worlds: how to solve real problems on modern quantum computers
Our work on hybrid quantum-classical algorithms is featured in news
The best of both worlds: how to solve real problems on modern quantum computers
Wednesday, June 19, 2019
Hybrid quantum-classical algorithms
Our paper on hybrid quantum-classical algorithms is featured in IEEE Computer, the June's issue on quantum realism.
Ruslan Shaydulin, Hayato Ushijima-Mwesigwa, Christian F.A. Negre, Ilya Safro, Susan M. Mniszewski, Yuri Alexeev "Hybrid Approach for Solving Optimization Problems on Small Quantum Computers", IEEE Computer, vol. 52(6), pp. 18-26, 2019
Solving larger-sized problems is an important area of research in quantum computing. Designing hybrid quantum-classical algorithms is a promising approach to solving this. We discuss decomposition-based hybrid approaches for solving optimization problems and demonstrate them for applications related to community detection.
Thursday, May 23, 2019
Solving network community detection problem on quantum computers
Accepted paper in Advanced Quantum Technology journal
Shaydulin, Ushijima-Mwesigwa, Safro, Mniszewski, Alexeev "Network Community Detection On Small Quantum Computers", 2019, preprint at https://arxiv.org/abs/1810.12484
In recent years, a number of quantum computing devices with small numbers of qubits have become available. A hybrid quantum local search (QLS) approach that combines a classical machine and a small quantum device to solve problems of practical size is presented. The proposed approach is applied to the network community detection problem. QLS is hardware‐agnostic and easily extendable to new quantum computing devices as they become available. It is demonstrated to solve the 2‐community detection problem on graphs of sizes of up to 410 vertices using the 16‐qubit IBM quantum computer and D‐Wave 2000Q, and compare their performance with the optimal solutions. The results herein demonstrate that QLS performs similarly in terms of quality of the solution and the number of iterations to convergence on both types of quantum computers and it is capable of achieving results comparable to state‐of‐the‐art solvers in terms of quality of the solution including reaching the optimal solutions.
Monday, May 13, 2019
Monday, May 6, 2019
Synthetic planar graph generation
Accepted paper in Applied Network Science journal
Chauhan, Gutfraind, Safro "Multiscale planar graph generation", 2019, preprint at https://arxiv.org/abs/1802.09617
The study of network representations of physical, biological, and social phenomena can help us better understand their structure and functional dynamics as well as formulate predictive models of these phenomena. However, due to the scarcity of real-world network data owing to factors such as cost and effort required in collection of network data and the sensitivity of this data towards theft and misuse, engineers and researchers often rely on synthetic data for simulations, hypothesis testing, decision making, and algorithm engineering. An important characteristic of infrastructure networks such as roads, water distribution and other utility systems is that they can be (almost fully) embedded in a plane, therefore to simulate these system we need realistic networks which are also planar. While the currently-available synthetic network generators can model networks that exhibit realism, they do not guarantee or achieve planarity. In this paper we present a flexible algorithm that can synthesize realistic networks that are planar. The method follows a multi-scale randomized editing approach generating a hierarchy of coarsened networks of a given planar graph and introducing edits at various levels in the hierarchy. The method preserves the structural properties with minimal bias including the planarity of the network, while introducing realistic variability at multiple scales.
Reproducibility: All datasets and algorithm implementation presented in this work are available at https://bit.ly/2CjOUAS
Monday, April 29, 2019
Designing scalable nonlinear support vector machines
Accepted paper in Machine Learning journal
Sadrfaridpour, Razzaghi, Safro, "Engineering fast multilevel support vector machines", 2019, preprint at https://arxiv.org/abs/1707.07657
The computational complexity of solving nonlinear support vector machine (SVM) is prohibitive on large-scale data. In particular, this issue becomes very sensitive when the data represents additional difficulties such as highly imbalanced class sizes. Typically, nonlinear kernels produce significantly higher classification quality to linear kernels but introduce extra kernel and model parameters which requires computationally expensive fitting. This increases the quality but also reduces the performance dramatically. We introduce a generalized fast multilevel framework for regular and weighted SVM and discuss several versions of its algorithmic components that lead to a good trade-off between quality and time. Our framework is implemented using PETSc which allows an easy integration with scientific computing tasks. The experimental results demonstrate significant speed up compared to the state-of-the-art nonlinear SVM libraries.
Reproducibility: our source code, documentation and parameters are available at https://github.com/esadr/mlsvm
Saturday, March 30, 2019
Finding influential nodes in networks with consumable resources
Accepted paper in Network Science journal
Ushijima-Mwesigwa, Khan, Chowdhury, Safro, "Centralities for Networks with Consumable Resources", 2019, preprint at https://arxiv.org/abs/1903.00642
Identification of influential nodes is an important step in understanding and controlling the dynamics of information, traffic, and spreading processes in networks. As a result, a number of centrality measures have been proposed and used across different application domains. At the heart of many of these measures lies an assumption describing the manner in which traffic (of information, social actors, particles, etc.) flows through the network. For example, some measures only count shortest paths while others consider random walks. This paper considers a spreading process in which a resource necessary for transit is partially consumed along the way while being refilled at special nodes on the network. Examples include fuel consumption of vehicles together with refueling stations, information loss during dissemination with error-correcting nodes, and consumption of ammunition of military troops while moving. We propose generalizations of the well-known measures of betweenness, random-walk betweenness, and Katz centralities to take such a spreading process with consumable resources into account. In order to validate the results, experiments on real-world networks are carried out by developing simulations based on well-known models such as Susceptible-Infected-Recovered and congestion with respect to particle hopping from vehicular flow theory. The simulation-based models are shown to be highly correlated with the proposed centrality measures.
Friday, March 29, 2019
Future of medicine - man or machine
Some thoughts about literature based discovery and our automated biomedical hypothesis generation tool MOLIERE for Clemson World Magazine
Saturday, February 9, 2019
Does it help to charge the electric cars at intersections?
Accepted paper in Computer-Aided Civil and Infrastructure Engineering
Khan, Khan Chowdhury, Safro, Ushijima-Mwesigwa "Wireless Charging Utility Maximization and Intersection Control Delay Minimization Framework for Electric Vehicles"
This study presents the Wireless Charging Utility Maximization (WCUM) framework, which aims to maximize the utility of Wireless Charging Units (WCUs) for electric vehicle (EV) charging through the optimal WCU deployment at signalized intersections. Furthermore, the framework aims to minimize the control delay at all signalized intersections of the network. The framework consists of a two‐step optimization formulation, a dynamic traffic assignment model to calculate the user equilibrium, a traffic microsimulator to formulate the objective functions, and a global Mixed Integer Non‐Linear Programming (MINLP) optimization solver. An optimization problem is formulated for each intersection, and another for the entire network. The performance of the WCUM framework is tested using the Sioux Falls network. We perform a comparative study of 12 global MINLP solvers with a case study. Based on solution quality and computation time, we choose the Couenne solver for this framework.
https://onlinelibrary.wiley.com/doi/abs/10.1111/mice.12439
Sunday, February 3, 2019
Predicting bariatric surgery outcomes
Accepted paper in Annals of Operations Research
Razzaghi, Safro, Ewing, Sadrfaridpour, Scott "Predictive models for bariatric surgery risks with imbalanced medical datasets"
Bariatric surgery (BAR) has become a popular treatment for type 2 diabetes mellitus which is among the most critical obesity-related comorbidities. Patients who have bariatric surgery, are exposed to complications after surgery. Furthermore, the mid- to long-term complications after bariatric surgery can be deadly and increase the complexity of managing safety of these operations and healthcare costs. Current studies on BAR complications have mainly used risk scoring for identifying patients who are more likely to have complications after surgery. Though, these studies do not take into consideration the imbalanced nature of the data where the size of the class of interest (patients who have complications after surgery) is relatively small. We propose the use of imbalanced classification techniques to tackle the imbalanced bariatric surgery data: synthetic minority oversampling technique (SMOTE), random undersampling, and ensemble learning classification methods including Random Forest, Bagging, and AdaBoost. Moreover, we improve classification performance through using Chi-squared, Information Gain, and Correlation-based feature selection techniques. We study the Premier Healthcare Database with focus on the most-frequent complications including Diabetes, Angina, Heart Failure, and Stroke. Our results show that the ensemble learning-based classification techniques using any feature selection method mentioned above are the best approach for handling the imbalanced nature of the bariatric surgical outcome data. In our evaluation, we find a slight preference toward using SMOTE method compared to the random undersampling method. These results demonstrate the potential of machine-learning tools as clinical decision support in identifying risks/outcomes associated with bariatric surgery and their effectiveness in reducing the surgery complications as well as improving patient care.
Monday, January 28, 2019
Saturday, January 12, 2019
Welcome new students!
Two new students, Zirou Qiu (MSc) and Korey Palmer (senior undergrad) are joining our research group.
Friday, January 11, 2019
Relaxation-Based Coarsening for Multilevel Hypergraph Partitioning
Accepted paper at SIAM Multiscale Modeling and Simulations
Ruslan Shaydulin, Jie Chen, Ilya Safro "Relaxation-Based Coarsening for Multilevel Hypergraph Partitioning", 2019, preprint at arXiv:1710.06552
Multilevel partitioning methods that are inspired by principles of multiscaling are the most powerful practical hypergraph partitioning solvers. Hypergraph partitioning has many applications in disciplines ranging from scientific computing to data science. In this paper we introduce the concept of algebraic distance on hypergraphs and demonstrate its use as an algorithmic component in the coarsening stage of multilevel hypergraph partitioning solvers. The algebraic distance is a vertex distance measure that extends hyperedge weights for capturing the local connectivity of vertices which is critical for hypergraph coarsening schemes. The practical effectiveness of the proposed measure and corresponding coarsening scheme is demonstrated through extensive computational experiments on a diverse set of problems. Finally, we propose a benchmark of hypergraph partitioning problems to compare the quality of other solvers.
Monday, November 26, 2018
Thesis defense
Congratulations to Varsha Chauhan for successfully defending her MSc thesis on planar graph generation!
Travel awards
Congratulations to Justin Sybrandt and Ruslan Shaydulin for receiving travel awards to present their papers at IEEE Big Data 2018 and APS 2018!
Thesis defense
Congratulations to Dr. Hayato Ushijima-Mwesigwa for successfully defending his Ph.D. thesis "Models for Networks with Consumable Resources"!
Community detection on NISQ devices
Accepted paper at at 3rd International Workshop on Post Moore's Era 2018
Supercomputing (PMES 2018)
Ruslan Shaydulin, Haayto Ushijima-Mwesigwa, Ilya Safro, Susan Mniszewski, Yuri Alexeev "Community Detection Across Emerging Quantum Architectures", preprint at arXiv:1810.07765, 2018
Sunday, November 25, 2018
Can we predict crimes in Chicago?
Our paper is accepted at IEEE Big Data 2018
Saroj K. Dash, I. Safro, Ravisutha S. Srinivasamurthy "Spatio-temporal prediction of crimes using network analytic approach", preprint at arXiv:1808.06241, 2018
It is quite evident that majority of the population lives in urban area today than in any time of the human history. This trend seems to increase in coming years. Studies say that nearly 80.7% of total population in USA stays in urban area. By 2030 nearly 60% of the population in the world will live in or move to cities. With the increase in urban population, it is important to keep an eye on criminal activities. By doing so, governments can enforce intelligent policing systems and hence many government agencies and local authorities have made the crime data publicly available. In this paper, we analyze Chicago city crime data fused with other social information sources using network analytic techniques to predict criminal activity for the next year. We observe that as we add more layers of data which represent different aspects of the society, the quality of prediction is improved. Our prediction models not just predict total number of crimes for the whole Chicago city, rather they predict number of crimes for all types of crimes and for different regions in City of Chicago.
What we do/Team/In news
Quantum Computing Quantum computers are expected to accelerate scientific discovery spanning many different areas such as medicine, AI, ...
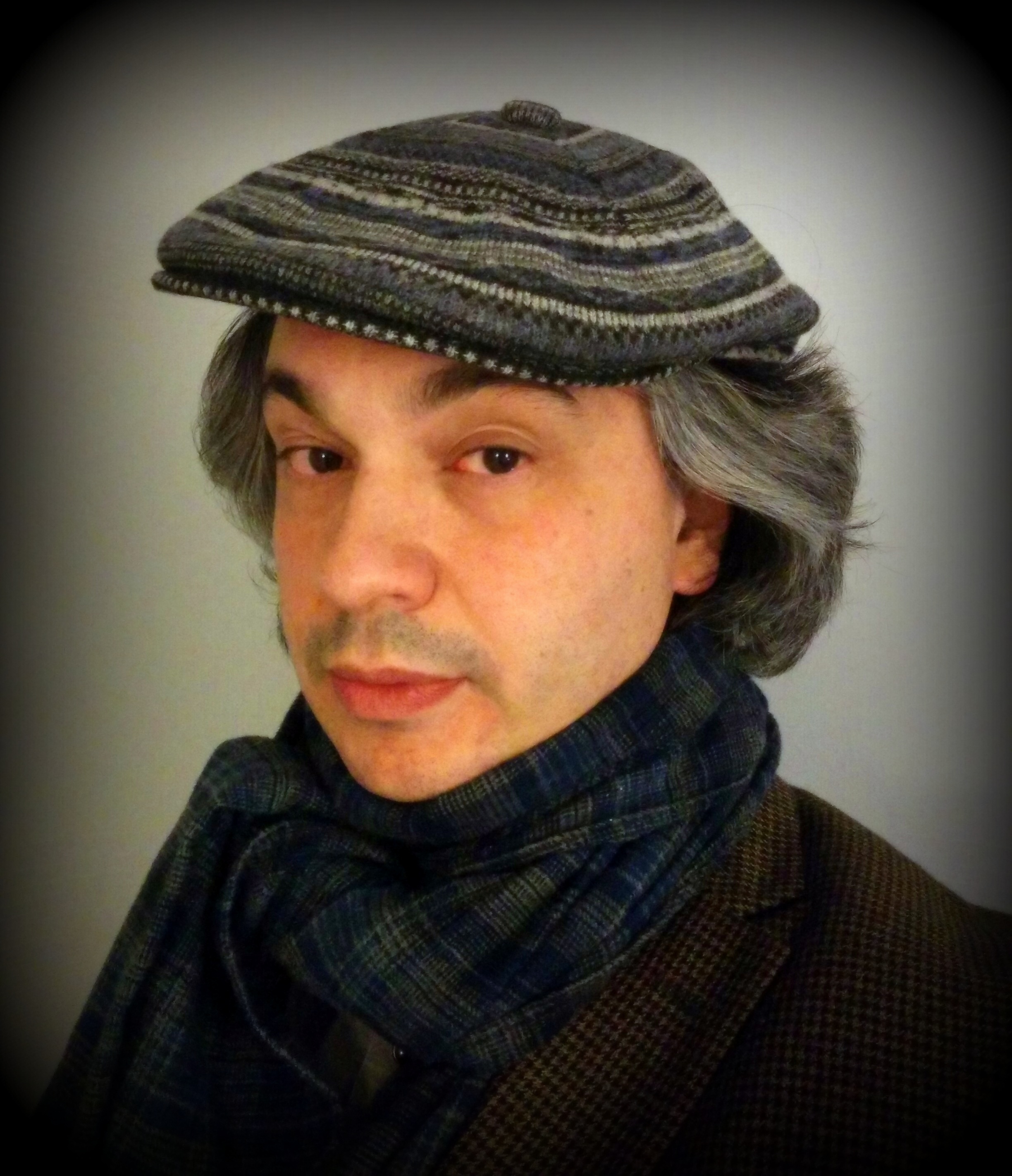
-
Quantum Computing Quantum computers are expected to accelerate scientific discovery spanning many different areas such as medicine, AI, ...
-
We're pleased to share that our group has expanded with the addition of two PhD students. Bao Bach, joining from the Quantum Science an...
-
We have another accepted paper which is the result of our collaboration with amazing colleagues at NASA/USRA, Rigetti and Purdue. Filip B. M...